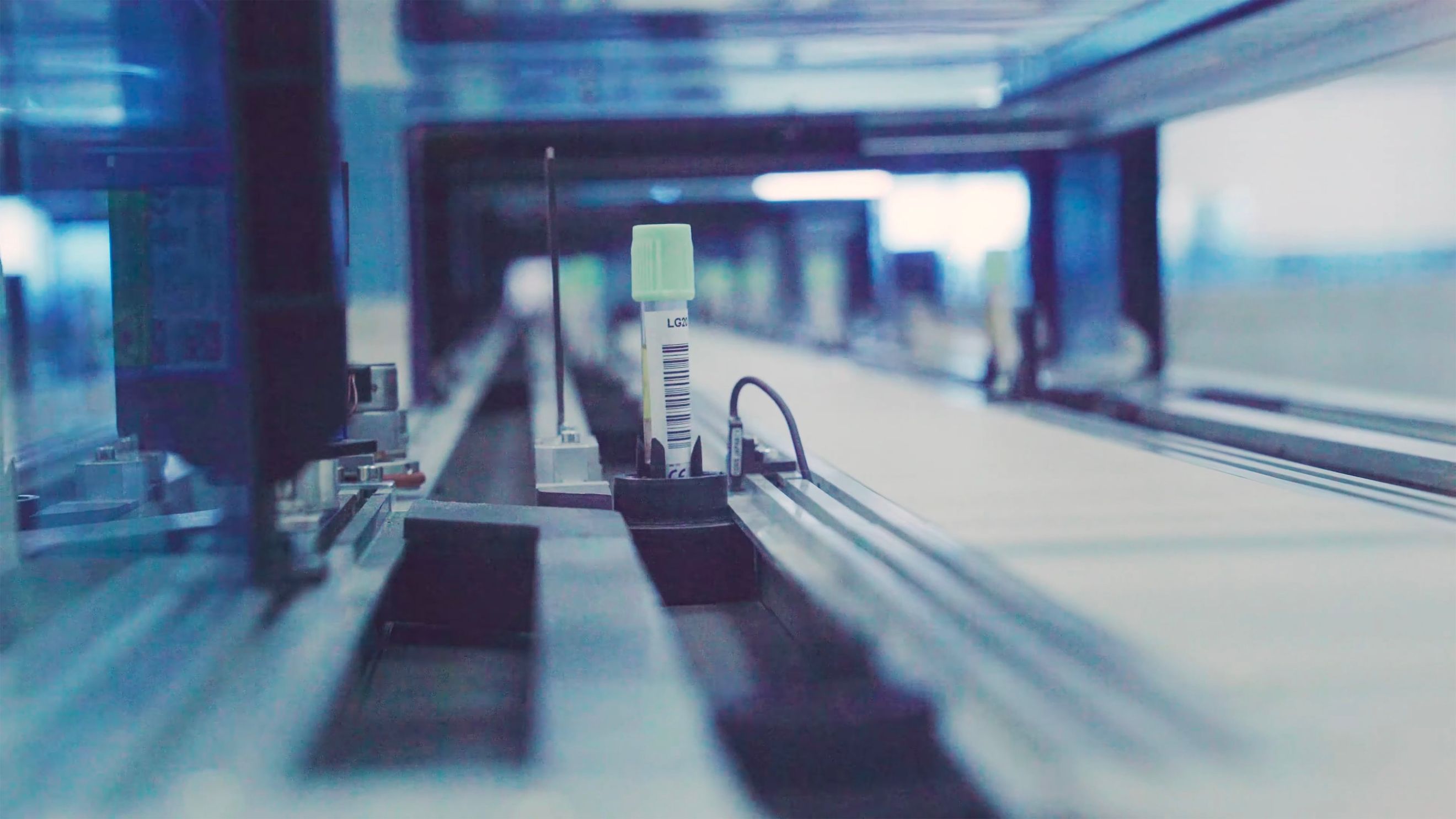
Deploy Zero-trust Diagnostic Assistant for Hospitals
Improving Hospital Diagnoses: How BlindAI and BastionAI Could Assist
While the benefits that AI could bring to hospitals could save lives, hospitals are still struggling to adopt these solutions due to privacy and security concerns. With BlindAI and BastionAI, image recognition tools hosted in the cloud can be used without exposing hospital data to any third party, unlocking new possibilities.
So how can we overcome the infrastructural challenges and privacy issues associated with deploying such AI by using Mithril's software, like BlindAI and BastionAI? This is the question we will explore in this article.
Key Takeaways:
- With BlindAI, your data is end-to-end protected, and you don't need to worry about the Adoption challenges of AI technologies
- Fortified Learning helps you deal with Data access and fine-tuning limitations
Introduction
People often worry about AI's efficiency and the broad consequences that come with it. But if there's one sector where everyone wants efficient tech solutions powered by AI… it's healthcare. Indeed, one study has shown that 80% of doctors would be more likely to use a medical device if it used AI.
One of the most sought-after goals of AI is to develop a machine to support doctors in identifying diseases. Diagnosis is still difficult for doctors and can be costly in terms of time and money. It is easy to imagine how consequences can be disastrous for patients when the diagnosis is incorrect or too slow a process.
Diagnosis is a complex task. First, it is essential: it is the beginning of care, and its accuracy and timeliness will significantly impact the success of the recovery. Diagnosis requires time: the anamnesis is extended and must be completed by numerous different exams (x-rays, specialist appointments, etc.), which leads to an increasingly lengthy diagnosis process. All of this contributes to a "strain" around the diagnosis.
Thus, in the USA, an estimated 40,000 to 80,000 people die annually from complications stemming from misdiagnoses.
AI-based diagnostic assistant solutions could allow a fast and accurate diagnosis by analyzing medical data: x-rays, pulse and respiration rate, etc…
Thanks to AIs, doctors would see their possibilities significantly increased, allowing them to diagnose patients more quickly and provide faster and safer support for patients.
For example, in South America, 600 deaths per year could be prevented by an earlier diagnosis of HIV. Our societies have a great need for more diagnoses.
However, while the demand for diagnosis is enormous, hospitals cannot meet it. They are overloaded; emergency rooms are faced with endless queues: helping doctors to make faster diagnoses would be a real game-changer. Medical diagnostic AI is, therefore, a real public health issue, and we intend to do our part to help doctors save lives.
In fact, we are not far from achieving this goal; the technologies already exist. The technology is efficient enough today to help save lives. Recent studies even show that AI makes better diagnoses than doctors on some diseases. Startups like Butterfly, Arterys, or Caption Health are already doing a great job! And there are so many more… In this field, each day sees the emergence of new solutions.
But the deployment of AI-based diagnostic assistants is still slow and sluggish. Despite their tremendous potential, AIs are still far from being commonplace in hospitals. So why is this?
Two main factors can explain this situation:
- The adoption of such technologies is immensely slow: the deployment is complex and very expensive. Moreover, the data's sensitivity can deter hospitals from moving data into the Cloud, preferring an on-prem deployment.
- The models' fine-tuning is time-consuming and could be improved with better access to data. Data is not readily available, which makes model training very difficult. The data used is highly sensitive, which limits its use, whether for security, compliance, or privacy reasons.
However, at Mithril Security, we are anything but defeatist. So how can we solve these issues and why should we? This is what we will explore in the following article.
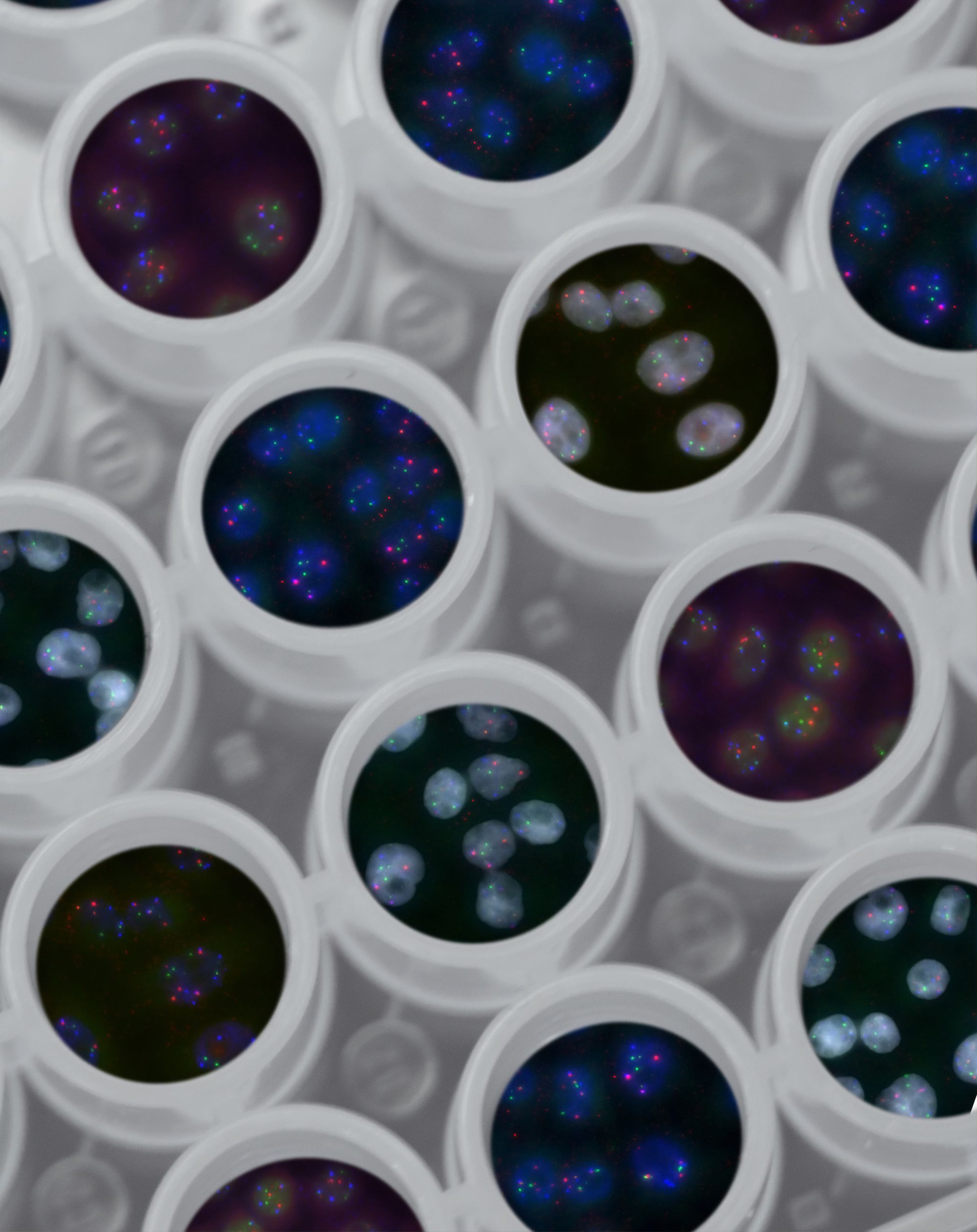
I. How to deal with the Adoption
The problem
As we have seen above, the potential of AI to help doctors save lives is enormous. But while many AIs have already been developed and are already working, their deployment is limited by infrastructure cost.
It's no secret that data centers represent a massive investment. Deploying those solutions, hardware, and software components is pretty complex and costly; They are so huge that hospitals cannot support them alone. And that's not all, opening up their IT system increases the risk of computer breaches - there is an increased risk of cyber attack - sending medical data outside the hospital is very risky for privacy - and can be punished by law in case of a leak… So there are many obstacles that lead hospitals to abandon AI.
Whilst AI saves lives, the benefits of scanners are more visible and direct. However, waiting too long for a diagnosis can also be harmful.
But there is a solution; you might point to sharing the costs of a data center by turning to the Cloud. Indeed, it is true that one natural way for companies to deploy, share or train their AI is through a Cloud Solution Provider. In addition, deploying their AI as a Service makes it easy to integrate with existing solutions and facilitates onboarding. Nonetheless, deploying such a solution in the Cloud creates privacy and security challenges. Respecting confidentiality is key to maintaining trust, but it is hard to provide guarantees that data will never be accessed.
Malicious insiders, such as a rogue admin, the Cloud provider, or even a hacker with internal access, could all compromise this extremely sensitive data.
Because of these non-negligible risks and the lack of privacy-by-design AI production tools, deploying this AI in the Cloud can become highly complex.
So it would appear that the magical solution of the Cloud is not suitable for hospitals. So are hospitals doomed to using expensive on-prem infrastructures? Or is there another way…
The solution
The solution we offer, at Mithril Security, is to unlock this tremendous opportunity but to unlock it safely. With BlindAI, the opportunity is that data is end-to-end protected. Thanks to TEE and enclaves, no third-party representative would be able to access the data you share in clear.
By leveraging secure enclaves, it becomes possible to guarantee data protection from end-to-end, even when we send data to a third party hosted in the Cloud.
We offer a solution to the problem of infrastructure costs through the Cloud with end-to-end protected data.
But to function, these AIs need data, and not just any data: very confidential data: medical X-rays, scans, samples, swabs, data such as pulse rate, white blood cell count, data relating to microbes or substances found in the body- in short, private data that no one would like to see in the public sphere.
Let's imagine you have a startup that has developed a state-of-the-art medical diagnostic assistant to support Doctors.
Enormous amounts of information lie in hospital databases. But those data are too sensitive. We are not talking about data like dog pictures; we are talking about medical data. This data can not be leaked because of how serious the implications of any malicious usage of the data could be.
This is the problem we solve with BlindAI. The Cloud is only a good solution to the infrastructure problem if it is secure and if hospitals can share their data with third parties with confidence.
With BlindAI, if those third parties are attacked and your data is compromised, if they are not trustworthy or reliable, it is not a problem because your data has been protected, and none of your patients would end up with their health data on the internet and Data Regulators could never sue you for not providing enough data security.
By using BlindAI, data can be sent to be analyzed by an AI in the Cloud without ever being revealed clearly to anyone else.
Therefore, hospitals can benefit from a state-of-the-art service without going through complex on-premise deployment and adopt a solution they do not need to constantly maintain, all the while keeping a high level of data protection as their data is not exposed to the Service Provider or the Cloud Provider.
Feel free to check out our article dedicated to BlindAI on our blog to learn more.
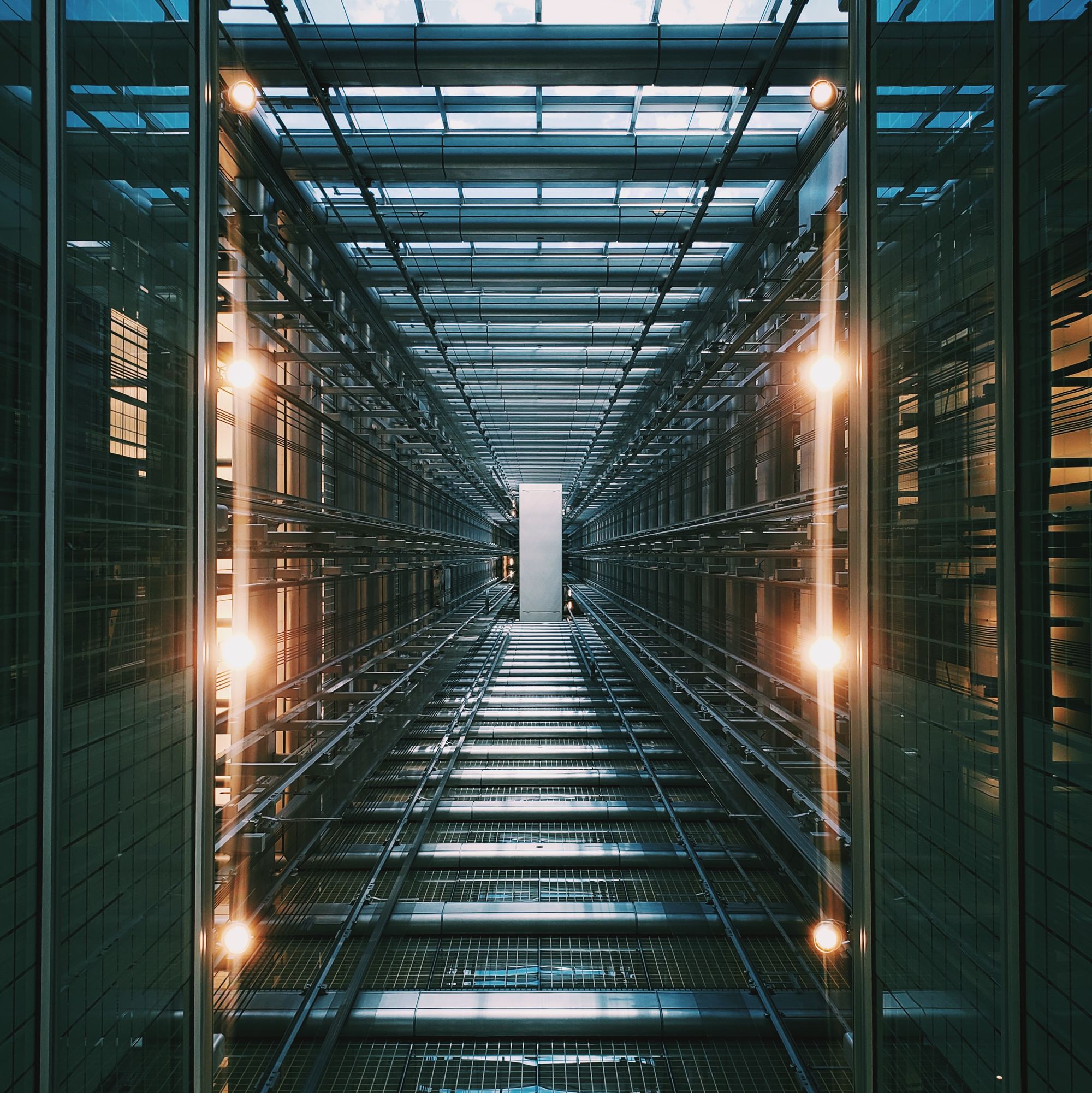
II. How to deal with the Data Access issues
The problem
The second problem we face when discussing diagnostic medical AIs is how to share and compile datasets securely given the sensitivity of the data they treat.
Let's take as an example the startups mentioned above: most of the time, they have been trained with the data from only one hospital. Currently, medical AI creators are limited by their customers' data. Therefore, each client will have its own AI trained from its dataset.
If these AI solutions are already having success with only one dataset, imagine the results with several datasets! It is a well-known fact: that more data provided is relevant, making better AI solutions. Moreover, this also eliminates AI bias. Because hospitals' datasets are very biased, they only apply to the population they treat.
One study showed that training an AI from a European and an Asian hospital dataset significantly improved its algorithms. Being trained from both Caucasian and Asian data resulted in an AI that was more useful in more situations. But, it is far from being commonplace.
So why are hospital datasets not being combined for better AI solutions? Because hospitals, to their credit, play it safe and refuse to share their precious data for AI model training, too afraid of the risk of misuse or theft.
This is a great loss for society; imagine the opportunities that would come with better AI solutions in the medical domain.
Hopefully, recent approaches based on federated learning, and unsupervised learning, have unlocked new horizons. This is the potential of multi-party learning: to get more versatile, accurate AI that could treat anyone. Nevertheless, to have this result, hospitals need to share their data. But you can’t simply buy medical data.
Medical AI models, like federated learning models, can be trained on huge amounts of sensitive data from hospitals. So how do they do it while complying with GDPR and other data regulations?
Well, the data never really leaves the hospitals so there is no risk of a leak and no regulation violation.
Unfortunately, those approaches - like federated learning - are slow and require heavy investments. This is primarily due to the extensive on-prem infrastructure necessary to comply with privacy laws.
But once again, at Mithril Security, we do not easily give in, and we intend to play our role in the democratization of medical diagnosis by AI.
The solution
At Mithril Security, we believe there is a third way: democratize privacy-friendly AI to help improve AI systems without compromising privacy or relying on complex on-prem infrastructure. That is why we have built BastionAI, an open-source and privacy-friendly solution to infer AI models with end-to-end protection.
BastionAI provides a way for hospitals to train confidential AI models to leverage AI without worrying that their data could be misused by anyone. As we have seen above, the technology is already there and works like a charm. It must be allowed to continue its development and reach its full potential.
Here is our proposal: allow AI models to be trained based on several datasets from different hospitals. Thus increasing their relevance, speed, and crucially their flexibility: they can diagnose any patient, even the rarest cases - because very diversified datasets have been used to train the models.
For this, we have developed a new technique called Fortified Learning. Here again, our solution goes through the Cloud. However, unlike Federated Learning, where there are several nodes, Fortified Learning has a central node in a secure enclave. This method dramatically decreases the required investments for those multi-party learning methods, especially for on-premises servers.
Thanks to this, the Fortified Learning method is faster than the Federated Learning one, with a high level of security and a frictionless experience. BastionAI is easy to use, easy to deploy, and can be really useful for researchers. Our goal is to offload most of the workload to a remote secure enclave. And thanks to the Cloud, BastionAI unlocks many new possibilities: the deployment is easy, costless, and scalable, all without compromising security or privacy.
Check out our article dedicated to BastionAI on our blog to learn more.
Get Involved
We welcome contributions to help us grow our confidential AI training framework. If you are interested in our technologies, you can test BlindBox directly, for free, according to our documentation. If you like what you see, drop us a star, contribute on GitHub, and reach out to us on our Discord!